
The era of artificial intelligence has dawned, with Generative AI emerging as a transformative force in revolutionizing everyday technology. An array of free AI tools now effortlessly crafts stunning images, texts, music, videos, and more in mere seconds. Remarkable platforms like Adobe’s AI Generative Fill in Photoshop and Midjourney’s innovations continue to astonish. But what precisely is Generative AI, propelling such swift innovation? Delve into our comprehensive guide to unravel the intricacies of Generative AI.
Definition: Decoding Generative AI
Generative AI, as the name suggests, refers to a type of AI technology capable of generating new content from trained data. It encompasses text, images, audio, videos, and synthetic data. This subfield of machine learning creates diverse outputs based on user input or prompts.
With a large training dataset, Generative AI can generate natural-sounding text combinations. The quality of output improves with the size of the dataset, particularly if it has been cleaned before training.
If you’ve trained a model with a large corpus of images, including tagging, captions, and visual examples, the AI can glean insights and excel at tasks like image classification and generation. This intricate AI system, designed to learn from examples, is known as a neural network.
Various types of Generative AI models exist, such as Generative Adversarial Networks (GAN), Variational Autoencoder (VAE), Generative Pretrained Transformers (GPT), Autoregressive models, and others. Below, we’ll provide a brief overview of these generative models.
Currently, GPT models, notably GPT-4/3.5 (ChatGPT), PaLM 2 (Google Bard), GPT-3 (DALL – E), LLaMA (Meta), Stable Diffusion, and others, have gained popularity. These user-friendly AI interfaces are all built on the Transformer architecture. Thus, in this explanation, our focus will primarily be on Generative AI and GPT (Generative Pretrained Transformer).
What Are the Different Types of Generative AI Models?
Among Generative AI models, GPT stands out, but let’s begin with GAN (Generative Adversarial Network). This architecture involves two parallel networks: one for content generation (generator) and the other for content evaluation (discriminator).
Essentially, the goal is to pit two neural networks against each other to produce results mirroring real data. GANs have primarily been utilized for image generation.
GAN (Generative Adversarial Network) / Source: Google
Next, we explore the Variational Autoencoder (VAE), which involves encoding, learning, decoding, and generating content. For instance, given an image of a dog, it analyzes features such as color, size, and ears, learns typical dog characteristics, then generates a simplified representation of the image. Subsequently, it enhances the image by adding more variety and nuances.

Exploring Autoregressive models, akin to the Transformer model yet without self-attention, primarily generates texts by predicting subsequent parts based on preceding sequences. Additionally, we’ll delve into Normalizing Flows and Energy-based Models. Finally, we’ll discuss Transformer-based models extensively.
Understanding Generative Pretrained Transformer (GPT) Models
Prior to the emergence of the Transformer architecture, Recurrent Neural Networks (RNNs) and Convolutional Neural Networks (CNNs) such as GANs and VAEs were widely utilized in Generative AI. In 2017, Google researchers published a groundbreaking paper titled “Attention is all you need” (Vaswani, Uszkoreit, et al., 2017) aimed at advancing Generative AI and facilitating the development of large language models (LLMs).
Subsequently, Google introduced the BERT model (Bidirectional Encoder Representations from Transformers) in 2018, which adopted the Transformer architecture. Concurrently, OpenAI unveiled its inaugural GPT-1 model also based on the Transformer architecture.
Source: Marxav / commons.wikimedia.org
The key ingredient in the Transformer architecture that made it a favorite for Generative AI? The paper rightly titled it self-attention, absent in earlier neural network architectures. This predicts the next word in a sentence using Transformer, closely attending to neighboring words to establish context and relationships.
Regarding the “pretrained” term in GPT, it means the model has already been trained on massive amounts of text data before applying the attention mechanism. Pre-training on data teaches sentence structures, patterns, facts, phrases, etc., enabling a good understanding of language syntax.
How Google and OpenAI Approach Generative AI?
Google and OpenAI both utilize Transformer-based models: Google in Bard and OpenAI in ChatGPT. However, they diverge in approach. Google’s PaLM 2 employs a bidirectional encoder, incorporating surrounding words to comprehend sentence context and generate all words simultaneously. Google’s strategy involves predicting missing words within a given context.
In contrast, OpenAI’s ChatGPT employs the Transformer architecture to predict the next word – sequentially. It’s a unidirectional model for crafting coherent sentences, continuing until completion. This could be why Google Bard generates text more rapidly than ChatGPT. However, both rely on the Transformer architecture to power their Generative AI interfaces.
Generative AI Applications
Generative AI finds broad utility beyond text, extending into realms encompassing images, videos, and audio. AI chatbots like ChatGPT, Google Bard, and Bing Chat harness its capabilities. Its versatility spans autocomplete, text summarization, virtual assistance, translation, and music generation. Examples abound, including Google MusicLM and Meta’s MusicGen.From DALL-E 2 to Stable Diffusion, Generative AI drives the creation of lifelike images from text descriptions. In video generation, platforms like Runway’s Gen-1, StyleGAN 2, and BigGAN utilize Generative Adversarial Networks to produce realistic content. Moreover, Generative AI powers 3D model generation, with notable models including DeepFashion and ShapeNet.Image Generated by Midjourney
Generative AI extends its utility beyond conventional boundaries. In drug discovery, it offers invaluable assistance by crafting innovative medications tailored to combat particular diseases. An exemplar in this realm is AlphaFold, an initiative by Google DeepMind. Moreover, Generative AI facilitates predictive modeling, enabling forecasts of forthcoming events in finance and meteorology.
Constraints of Generative AI
Generative AI possesses immense capabilities but isn’t without failings. It necessitates a large corpus of data for model training, posing challenges for small startups with limited access to high-quality data. Notably, companies like Reddit, Stack Overflow, and Twitter have restricted access to their data or imposed high fees for it. Recently, The Internet Archive reported an hour of website inaccessibility due to an AI startup aggressively scraping its data for training.
Furthermore, Generative AI models face criticism for lack of control and inherent biases. Models trained on skewed internet data can disproportionately represent certain demographics, as seen in AI-generated images predominantly depicting lighter skin tones. Additionally, there’s the significant concern of deepfake video and image creation using Generative AI. As previously mentioned, these models lack comprehension of context and repercussions, often reproducing content based solely on training data.
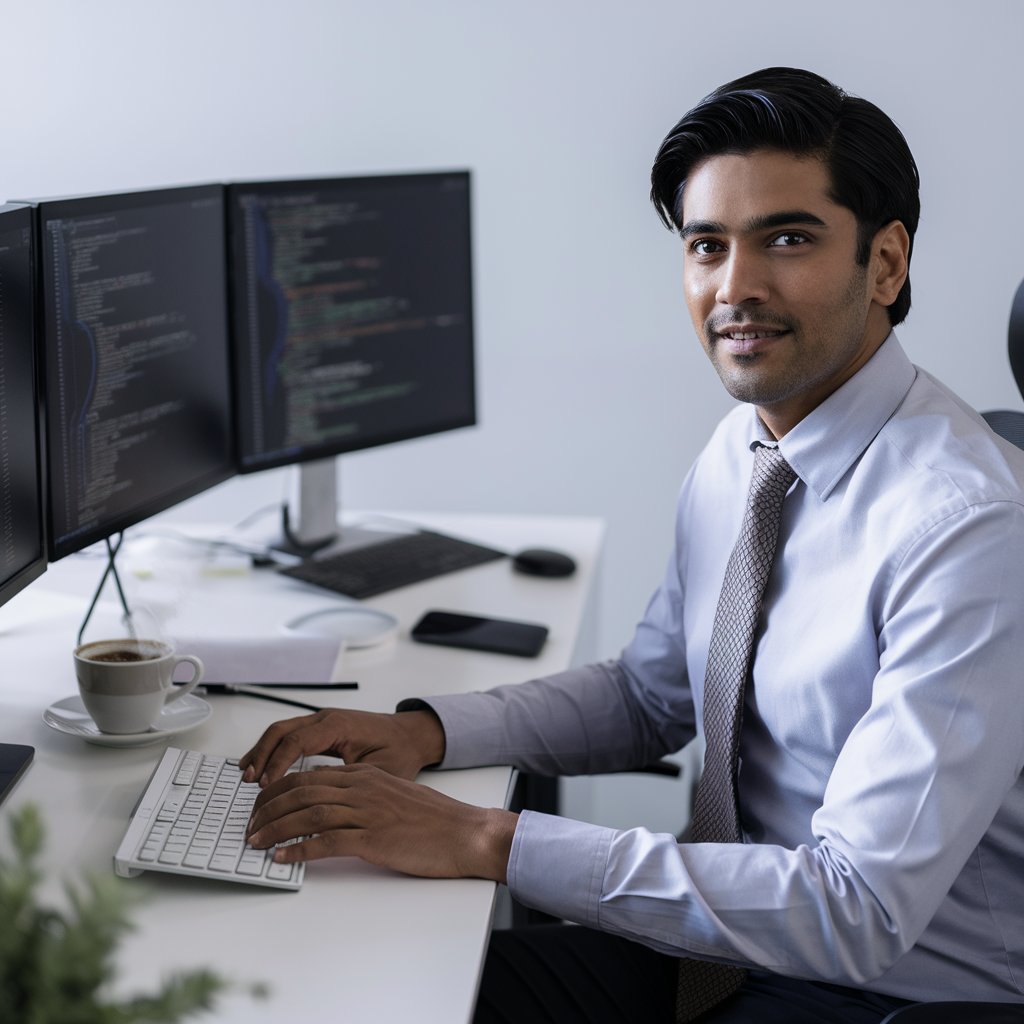
Pritam Chopra is a seasoned IT professional and a passionate blogger hailing from the dynamic realm of technology. With an insatiable curiosity for all things tech-related, Pritam has dedicated himself to exploring and unraveling the intricacies of the digital world.